The Future of Heart Failure Care: AI and Predictive Medicine
Early Detection, Personalized Treatments, and Improved Outcomes using AI
This post explores the integration of Artificial Intelligence (AI) in predictive medicine, with a focus on heart failure. Early detection of heart failure can be achieved through the analysis of chest X-rays using AI algorithms. Personalized treatment plans can also be created through the application of machine learning algorithms to patient data, leading to improved patient outcomes.
The integration of technology into healthcare has brought about significant advancements in patient outcomes and operational efficiency. One of the latest innovations in this field is the integration of Artificial Intelligence (AI). The clinical use of AI has rapidly increased over the years, from almost nonexistent in 2015 to over 30% in 2020, as reported by the American College of Radiology. Predictive medicine, a subset of AI in healthcare, is particularly promising in addressing heart failure, one of the leading causes of death and hospitalization worldwide.
Heart failure is a complex condition characterized by the heart's inability to pump sufficient blood to meet the body's needs. Despite advances in medical treatment and management, heart failure continues to be a significant public health concern, with a high rate of hospitalization and mortality. Predictive medicine, by leveraging patient data, has the potential to identify individuals at risk of heart failure and personalize treatments, ultimately improving patient outcomes.
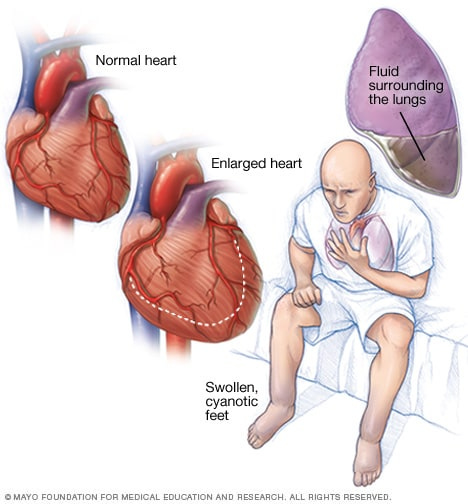
Early Diagnosis of Heart failure
Early detection of heart failure is crucial for improving patient outcomes and reducing mortality rates. Heart failure is a progressive disease that can lead to a decline in the patient's health and quality of life. By detecting the onset of heart failure at an early stage, patients can receive prompt treatment and lifestyle modifications, which can help slow down the progression of the disease. This, in turn, can reduce the number of hospitalizations, readmissions, and the costs associated with managing heart failure.
Chest X-rays are a commonly used diagnostic tool in many medical settings and they provide essential information about the heart and lungs. They are a low-cost, non-invasive, and readily available method for assessing the health of these vital organs. By using artificial intelligence algorithms, these X-rays can be scanned and analyzed to detect cardiomegaly (an enlarged heart) and pleural effusion (fluid accumulation around the lungs), which are both common signs of heart failure.
The "ART-IN-HF" project, a collaboration between Mersin University in Turkey and Qure.ai, scanned anonymous chest X-rays of patients who were at least 45 years old and applied to any department of the hospital, excluding cardiology, cardiovascular surgery and emergency departments. The AI detected patients who had both cardiomegaly (enlarged heart) and pleural effusion (fluid accumulation around the lungs) in their X-rays, two common signs of heart failure. Out of the 5623 subjects who were scanned, 2.1% (119) of them had both signs. Of the 119 patients, 57 were reached and 49 of them (86%) were diagnosed with heart failure according to the 2021 ESC HF guidelines.
This project demonstrates the power of predictive medicine in early disease detection and highlights the importance of using AI in routine medical assessments. By scanning X-rays for signs of heart failure, patients who may not have otherwise suspected they had the condition were identified and received prompt and effective treatment. The ability to detect diseases in their early stages, before they become severe and harder to treat, is a key aspect of predictive medicine.
Personalized Prognostication of Heart failure
Prognostication, or the ability to predict future health outcomes, is critical in the management of heart failure (HF). Accurate prognostication enables healthcare providers to make informed decisions about patient care and tailor treatment plans to best meet each individual's needs. Additionally, improved prognostication has the potential to inform the design of clinical trials and ultimately lead to the development of more effective therapies for HF. Current approaches to HF management have treated it as a singular disease, leading to inadequate patient care and inefficient clinical trials.
In a study in collaboration with Yale University School of Medicine, we applied machine learning algorithms to the Swedish Heart Failure Registry, a national registry of 44,886 heart failure patients, to identify predictors of 1-year survival. The results showed that random forest modeling demonstrated excellent calibration and discrimination for survival, whereas left ventricular ejection fraction did not. Cluster analysis revealed 4 clinically relevant subgroups of heart failure patients with marked differences in 1-year survival.
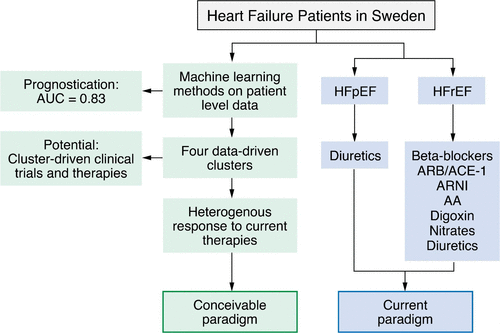
The study demonstrated significant interactions between the propensity-matched clusters and specific medications, indicating the potential for a more personalized approach to heart failure treatment. This study highlights the importance of machine learning and advanced analytics in predicting outcomes and improving the effectiveness of current therapies. The use of these novel analytic approaches has the potential to revolutionize the way heart failure is diagnosed and treated, leading to improved outcomes for patients.
Conclusion
The integration of artificial intelligence (AI) in predictive medicine is set to bring about significant improvements in the healthcare industry. Eric Topol, a renowned expert in the field, highlights that the problem with AI medical research is the notion of "man versus machine," instead of seeing the combination of man and machine as the solution. The integration of AI in predictive medicine has the potential to revolutionize healthcare by enabling early detection of diseases, personalized treatments, and improved patient outcomes. Our work in heart failure underscores our commitment towards this direction.